Event Streaming
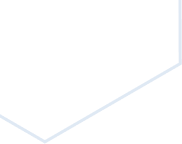
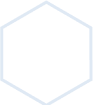
Event Streaming
Basis for real-time
Stackable for Event Streaming
The Stackable Data Platform serves as the basis for modern event streaming architectures and integrates seamlessly into Kubernetes. By using its own operators and integrating a number of curated open source data apps, Stackable offers a robust and flexible basis for processing and analyzing real-time data.
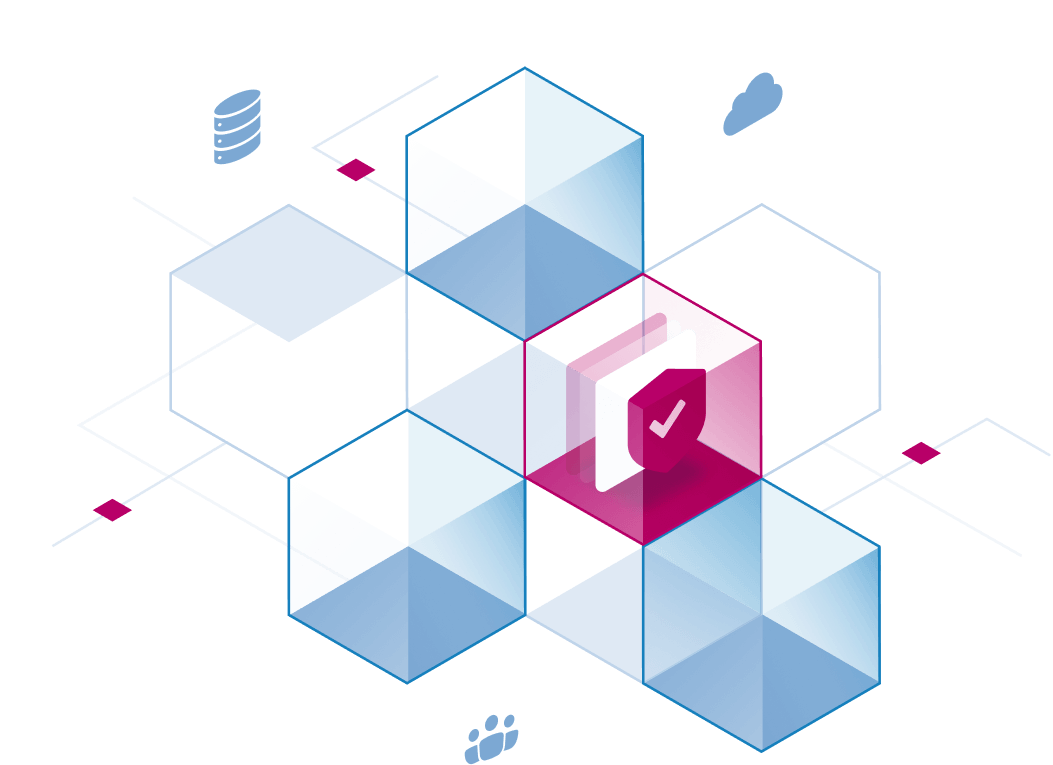

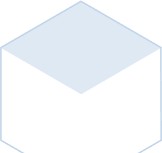
Event Streaming
Event Streaming is a dynamic method of handling data, enabling continuous processing and movement of data in real-time across systems. It’s crucial for organizations that require immediate insights and responses from their data, such as detecting fraudulent transactions, monitoring user activity in real-time, or managing supply chain logistics.
Highlighed data apps from the Stackable Data Platform for Event Streaming
Facilitates ingestion, data flow management and automated data exchange between systems.
- Easy Data Routing and Transformation: Offers a user-friendly interface for data flow management, supporting rapid design and deployment of processing pipelines.
- System Integration: Connects to a variety of data sources and sinks, facilitating data ingestion from disparate systems.
- Data Lineage: Tracks data flow from source to destination, enhancing auditing and compliance.
- Flexibility: Customizable processors and the ability to handle various data formats and sizes.
At the heart of our event streaming architecture, providing robust, scalable messaging and stream processing.
- High Throughput: Capable of handling millions of messages per second, making it ideal for large-scale message processing tasks.
- Scalability: Easily scales out with minimal downtime, supporting growing data needs.
- Stability and Reliability: Ensures data is not lost and can withstand failures, maintaining data integrity.
- Versatility: Supports a wide range of use cases.
Offers powerful stream processing capabilities, enabling complex analytics and machine learning on streaming data.
- In-Memory Computing: Accelerates processing speeds by keeping data in RAM, significantly faster than disk-based alternatives.
- Advanced Analytics: Supports complex algorithms for machine learning, graph processing, and more.
- Fault Tolerance: Resilient distributed datasets (RDDs) provide fault tolerance through lineage information.
- Language Support: Offers APIs in Python, Java, Scala, and R, broadening its accessibility and usability.
Provides real-time analytics and OLAP querying capabilities, ideal for insights on streaming data.
- Real-Time Analytics: Designed for sub-second query response times, making it ideal for interactive applications.
- Scalable: Handles massive volumes of data and concurrent users without compromising performance.
- High Availability: Distributed architecture ensures that the system is always on and can serve queries even during partial failures.
- Time Series Data: Optimized for ingesting and querying time-series data, perfect for metrics, monitoring, and event data.
Exemplary Use Case Scenarios
- Real-time Analytics and Monitoring: Implement Apache Kafka to capture and stream data, using Apache Spark for processing, enabling instant insights into business operations, customer behavior, and system performance.
- Fraud Detection: Utilize event streaming to continuously analyze transactions in real-time with Apache Kafka and Apache Spark, identifying and alerting on potential fraudulent activity, significantly reducing the risk and impact on businesses.
- Supply Chain Management: Streamline operations by monitoring supply chain events in real-time. Apache NiFi can be used for data collection and routing, while Apache Kafka streams the data, allowing for immediate adjustments to e.g. inventory levels, shipping schedules, and production plans.
- IoT Data Management: Manage vast streams of data from IoT devices with Apache Kafka and process with Apache Spark. Use Apache Druid for real-time analytics to monitor device health, optimize performance, and predict maintenance needs.
- Personalized Customer Experiences: Aggregate and process user activity and behavior data in real-time to provide personalized content, recommendations, and services. Use Apache Kafka for data streaming, Apache Spark for processing.
Showcase
This demo of the Stackable Data Platform shows streamed earthquake data up to the dashboard.
It uses Apache Kafka®, Apache Nifi, Apache Druid and also Apache Superset for visualization.
Our specialist for event streaming
Need more Info?
Contact Lars Francke to get in touch with us:
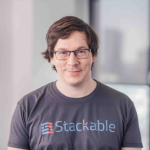
Lars Francke
CTO & Co-Founder of Stackable
Subscribe to our Newsletter
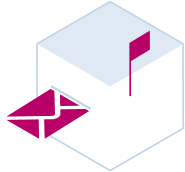
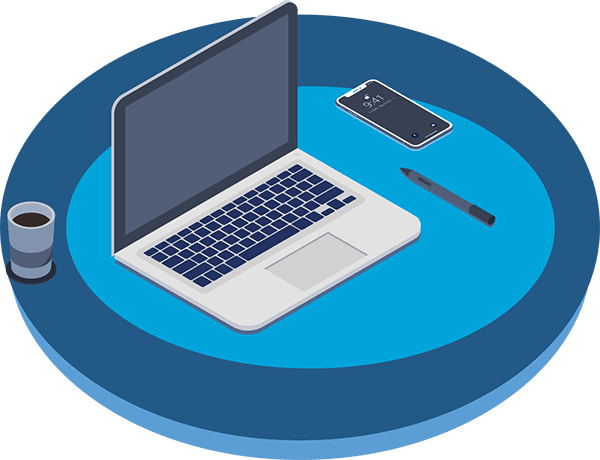
Newsletter
Subscribe to the newsletter
With the Stackable newsletter you’ll always be up to date when it comes to updates around Stackable!